FBAR Cards Insight
Fbar Cards is a card game designed to help researchers gain initial insights on informative hypothesis testing for ANOVA models as implemented in the 'restriktor' R package. The game goes like this:
- Set the "difficulty level" n
- Click Start/Restart Game to deal an n x n grid of cards from a standard 52 card deck
- For each row you can either:
- Leave the cards as is
- Swap two cards within the row
- ONLY 1 swap is allowed per row
- Click Score Game
In this game, the columns represent groups for an ANOVA analysis using an informative hypothesis testing framework where:
'col1 < col2 < col3'
is the constraint. As such, the user is expected to use their moves in each row to try to order the cards in increasing order.
Here is a sample hand of the game on level n = 3. After I click Start/Restart Game, I have the following grid:
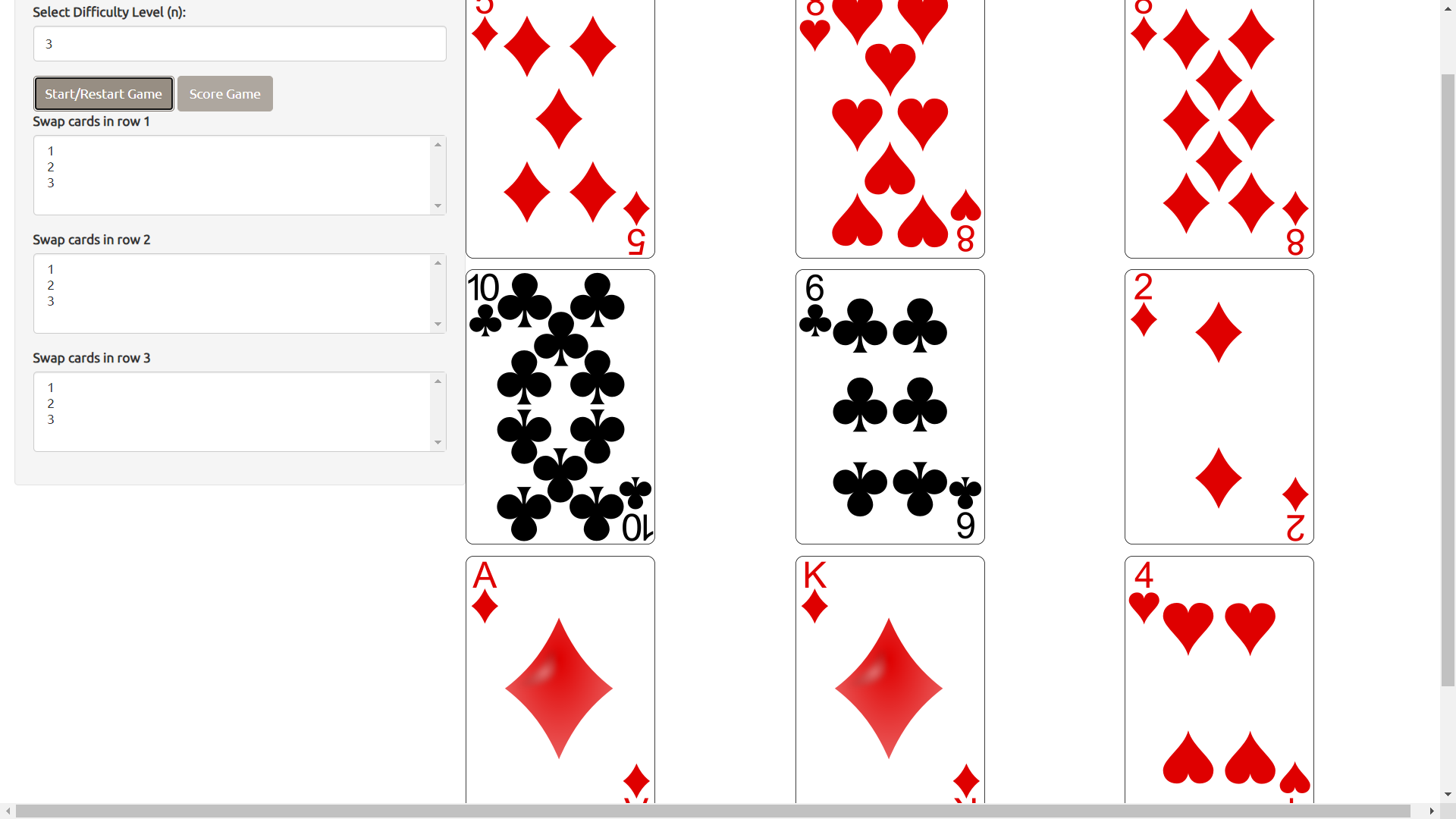
With this hand I made the following moves:
- Row 1: I did nothing in row 1. This was a mistake on my part; I forgot that hearts is defined as a higher value than diamonds. See the standard_deck function of 'mmcards' to see how the deck was defined (clubs < diamonds < hearts < spades).
- Row 2: Swapped 10 and 2
- Row 3: Swapped Ace and 4
This was the final hand:
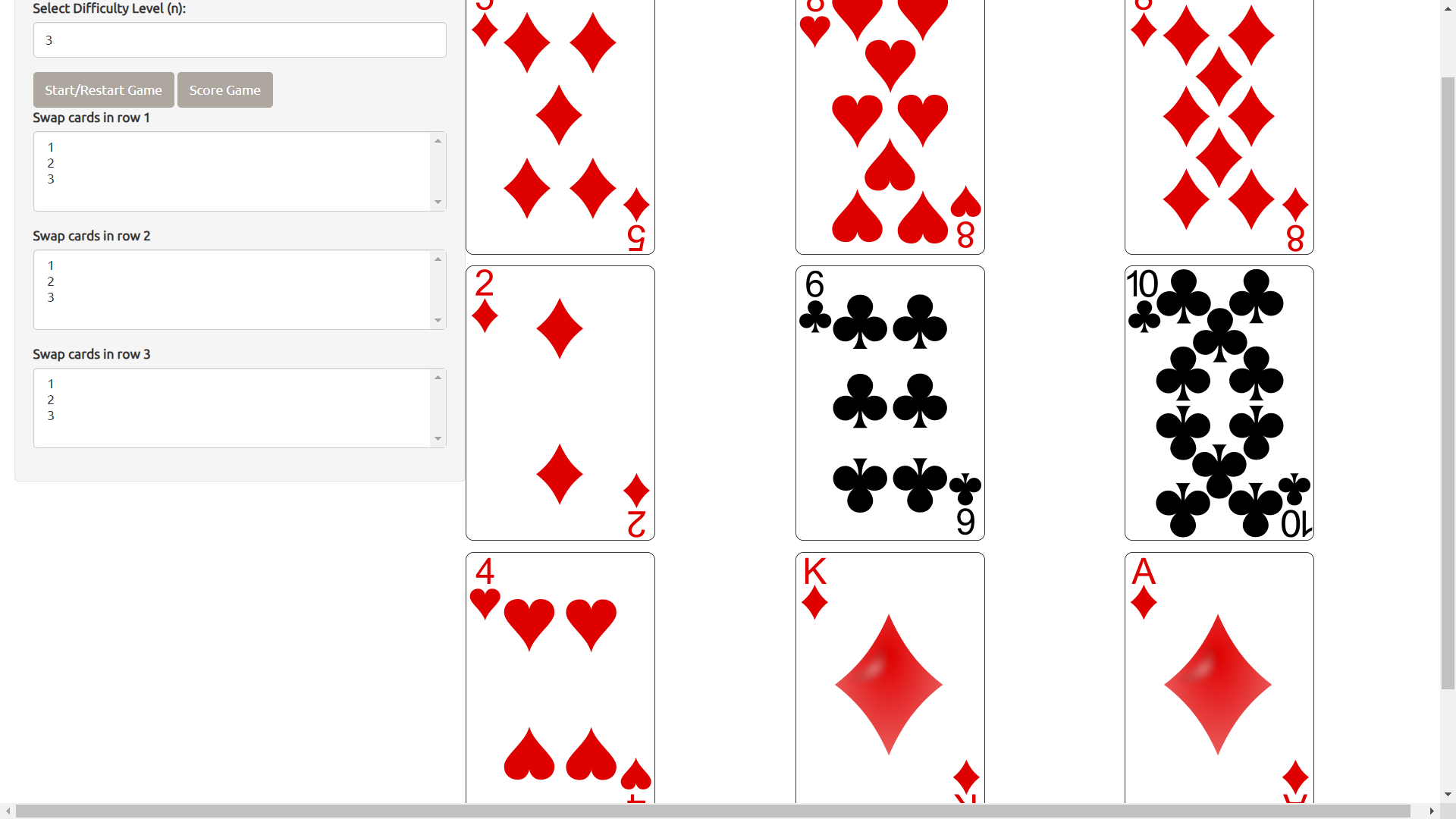
With this hand, I then clicked Score Game and obtained:
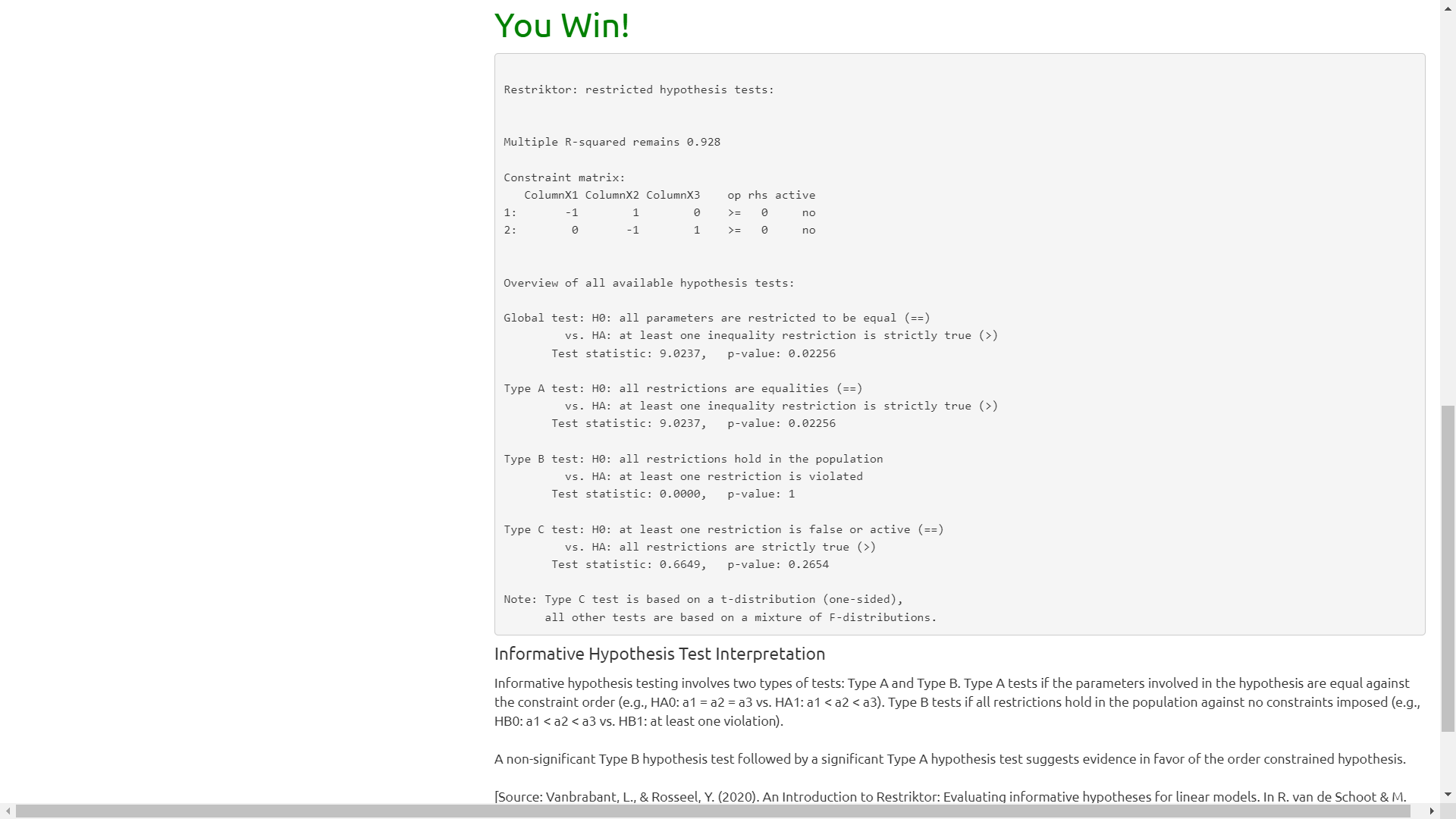
At this point, we can get into the insight.
Insight: Focus on the Edges
Since I'm only allowed one swap per row, when I play at higher difficulty levels, my main (and often only) focus is on making sure the card in column n has the largest value in the row. I've found that this strategy is surprisingly efficient.
In the paper Constrained statistical inference: sample-size tables for ANOVA and regression I came across a small passage which helps me understand what is going on here:
"In particular we would like to know about the power when the means are not perfectly in line with the ordered hypothesis
...
the power for Hypothesis test Type A (HA0 vs. HA1) is largely dominated by the extremes (here the first and last mean). This means that, irrespective of the deviations of the two middle means, the power is almost not affected."
Keep in mind that to "Win" we need to fail to reject the null hypothesis on the Type B test and reject the null hypothesis on the Type A test.
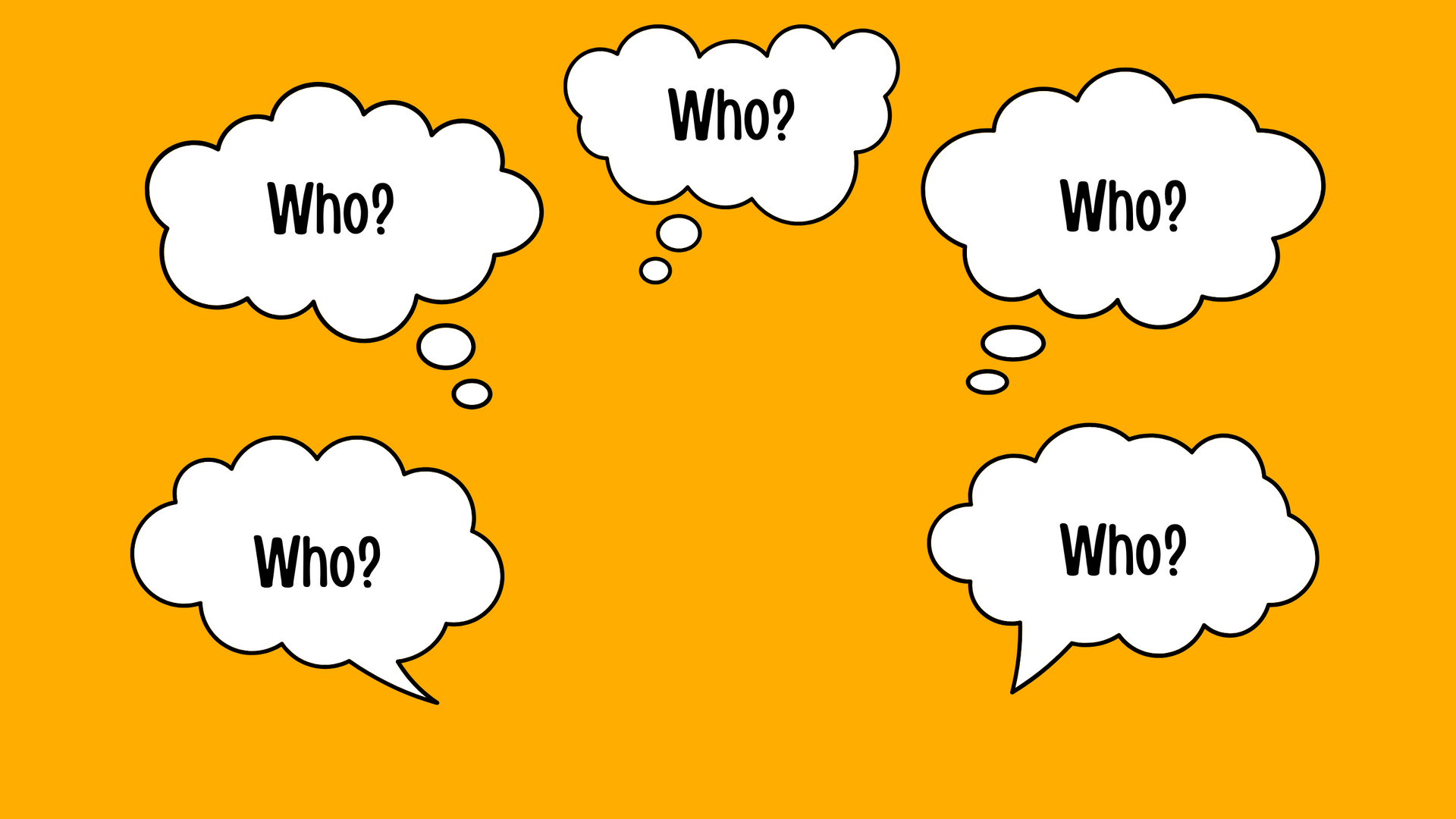
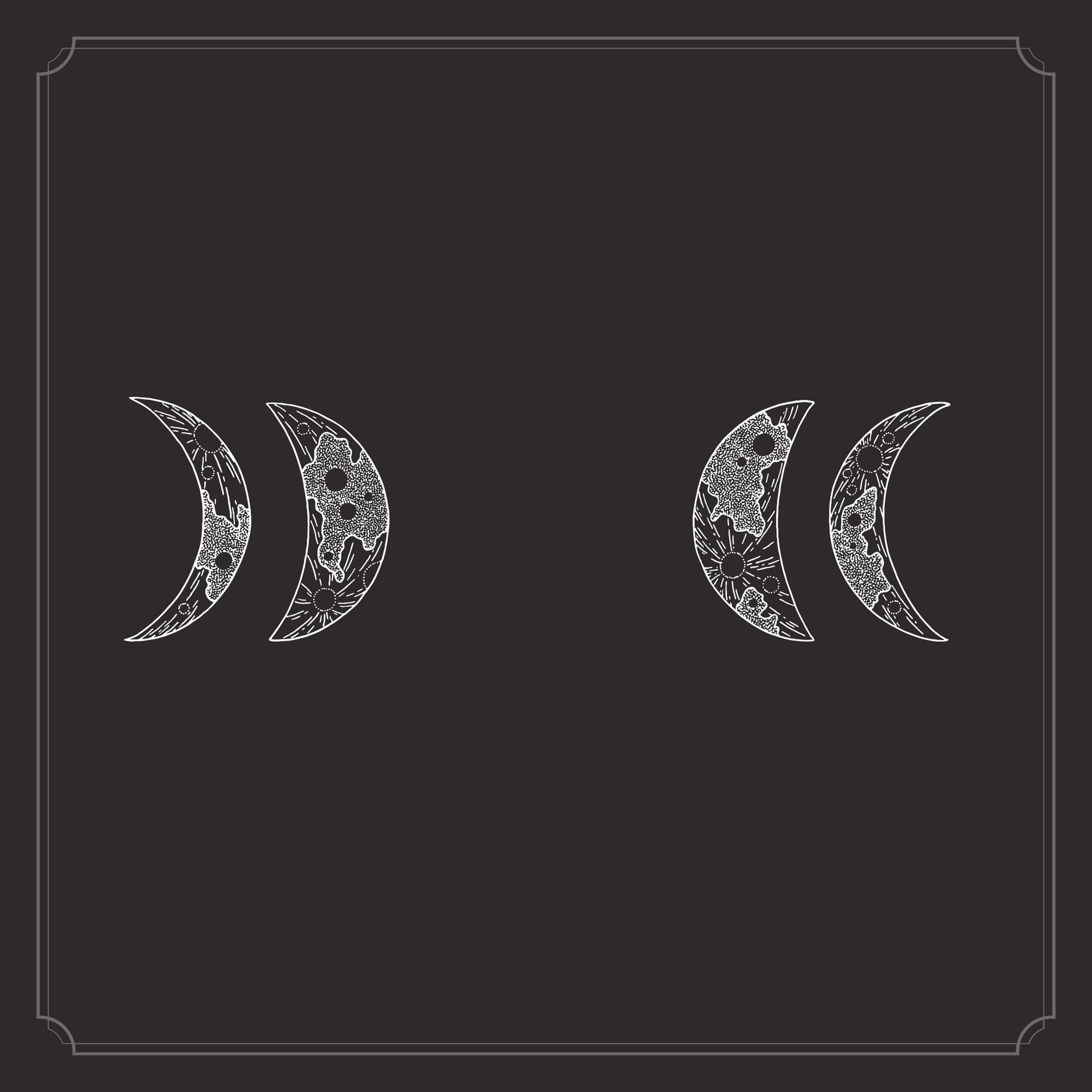
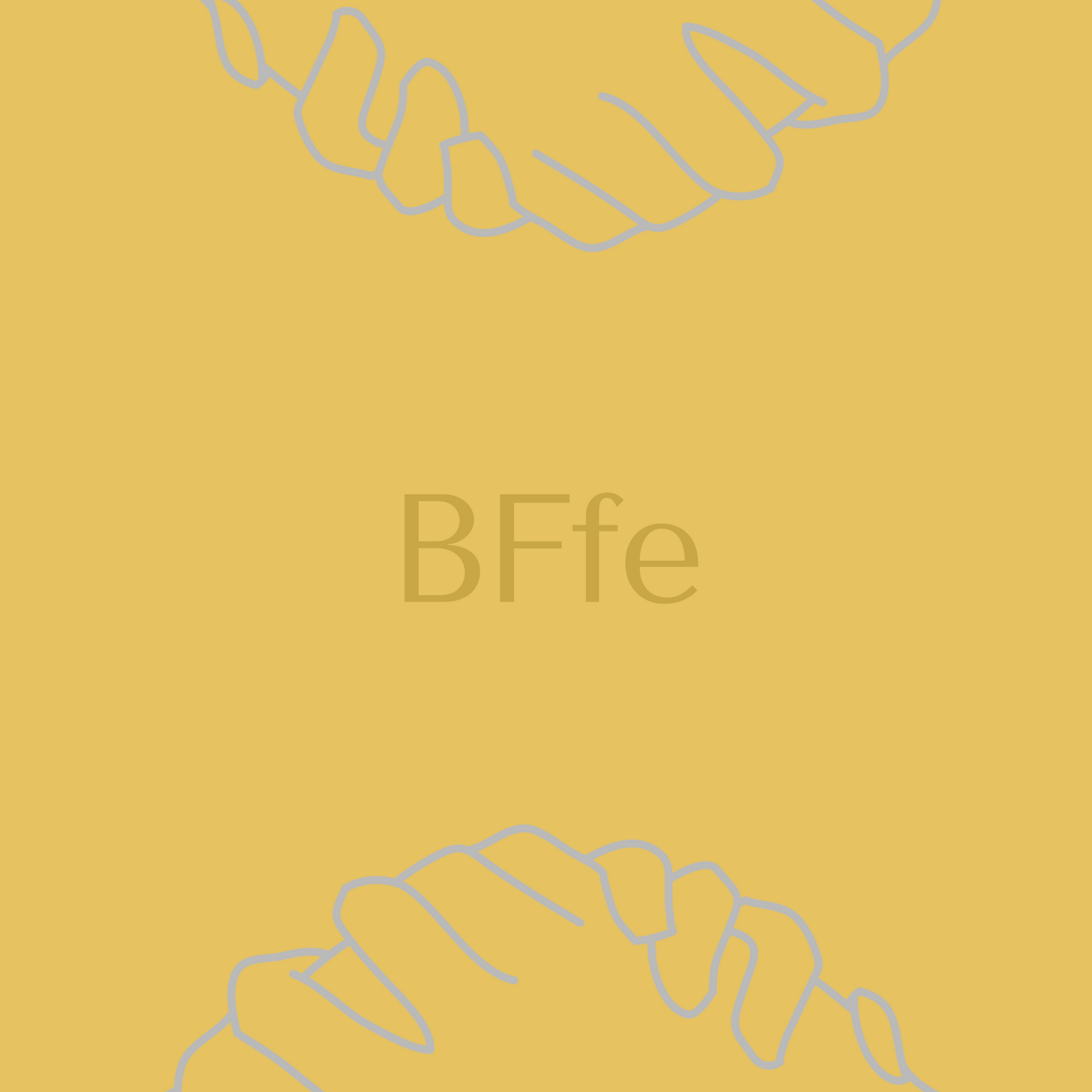